
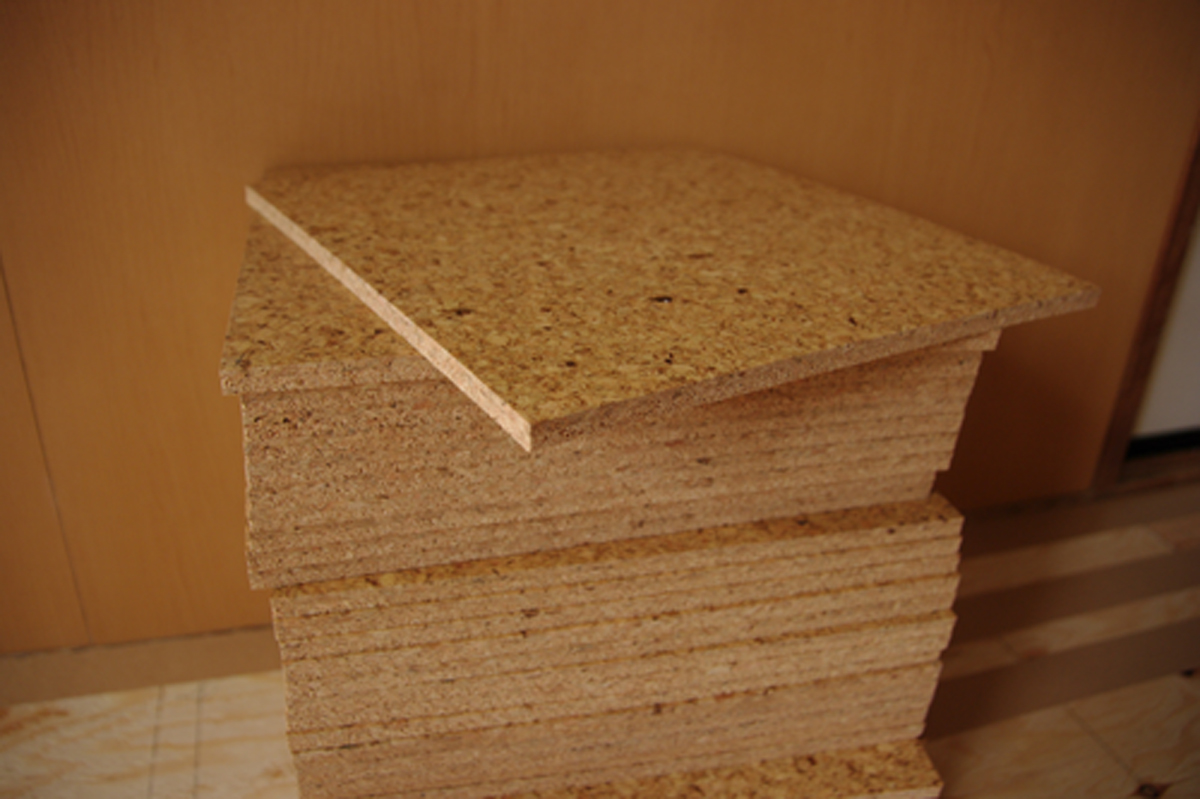
In Geometry, check Thin Walled for the SSS to be rendered as Translucency (Paper Shader). Subsurface Weight must be higher than 0.0 for the effect to be computed. To create a Translucent shader with Arnold: * Note that this option is suitable mainly for polygon surfaces without thickness (just one side) (the effect traditionally called Translucency or ‘Paper Shader’)
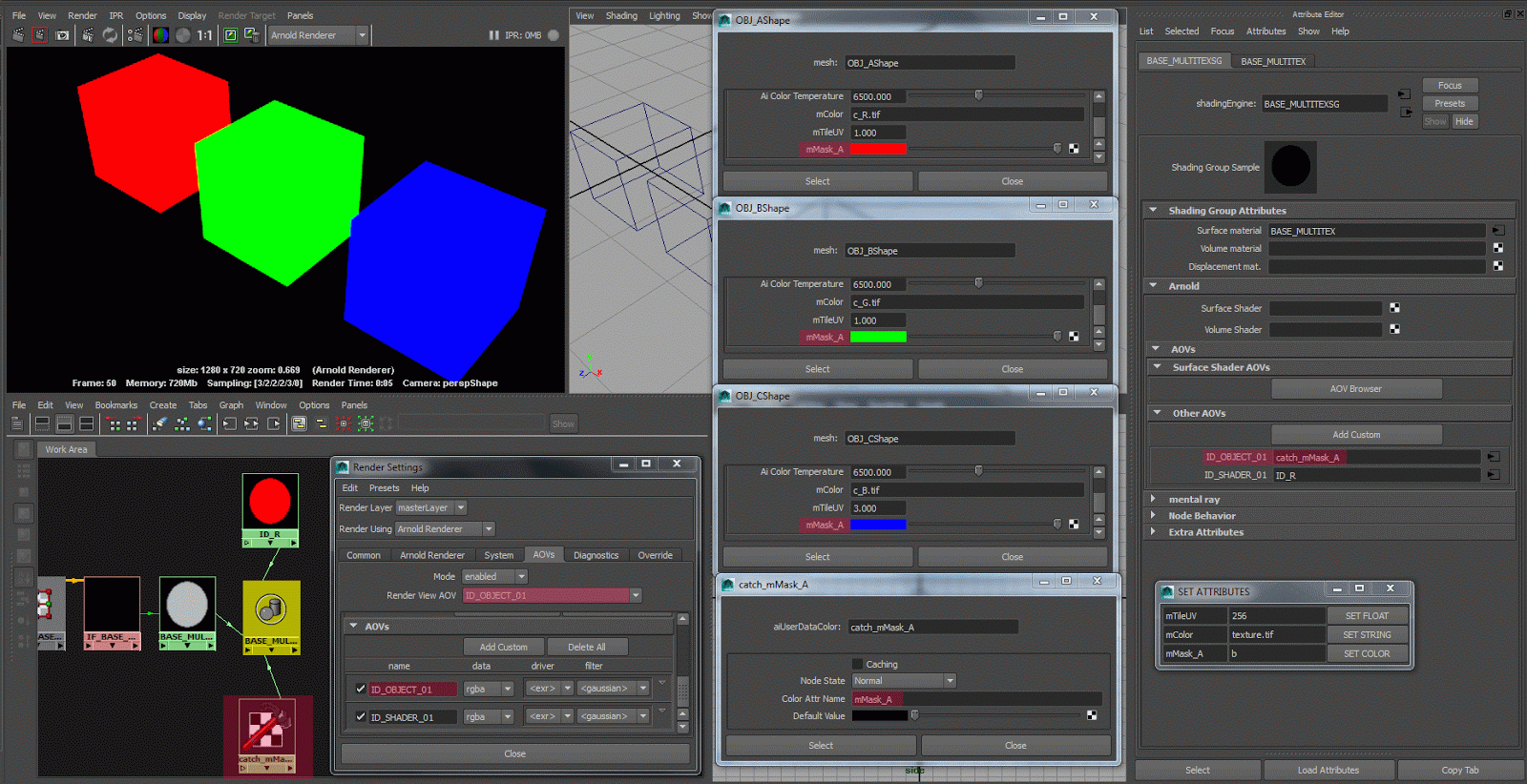
When the ‘Thin Walled’ option is checked in the Geometry attributes of the shader, the Subsurface isn’t rendered as a full volume of material like soap or skin/flesh (the effect that is traditionally called Subsurface Scattering – SSS) but as a thin paper-like translucent surface like paper, thin cloth thin leaves, lamp shades etc. The Subsurface component of the Arnold Standard Surface shader (aiStandardSurface) controls Sub Surface Scattering (SSS).
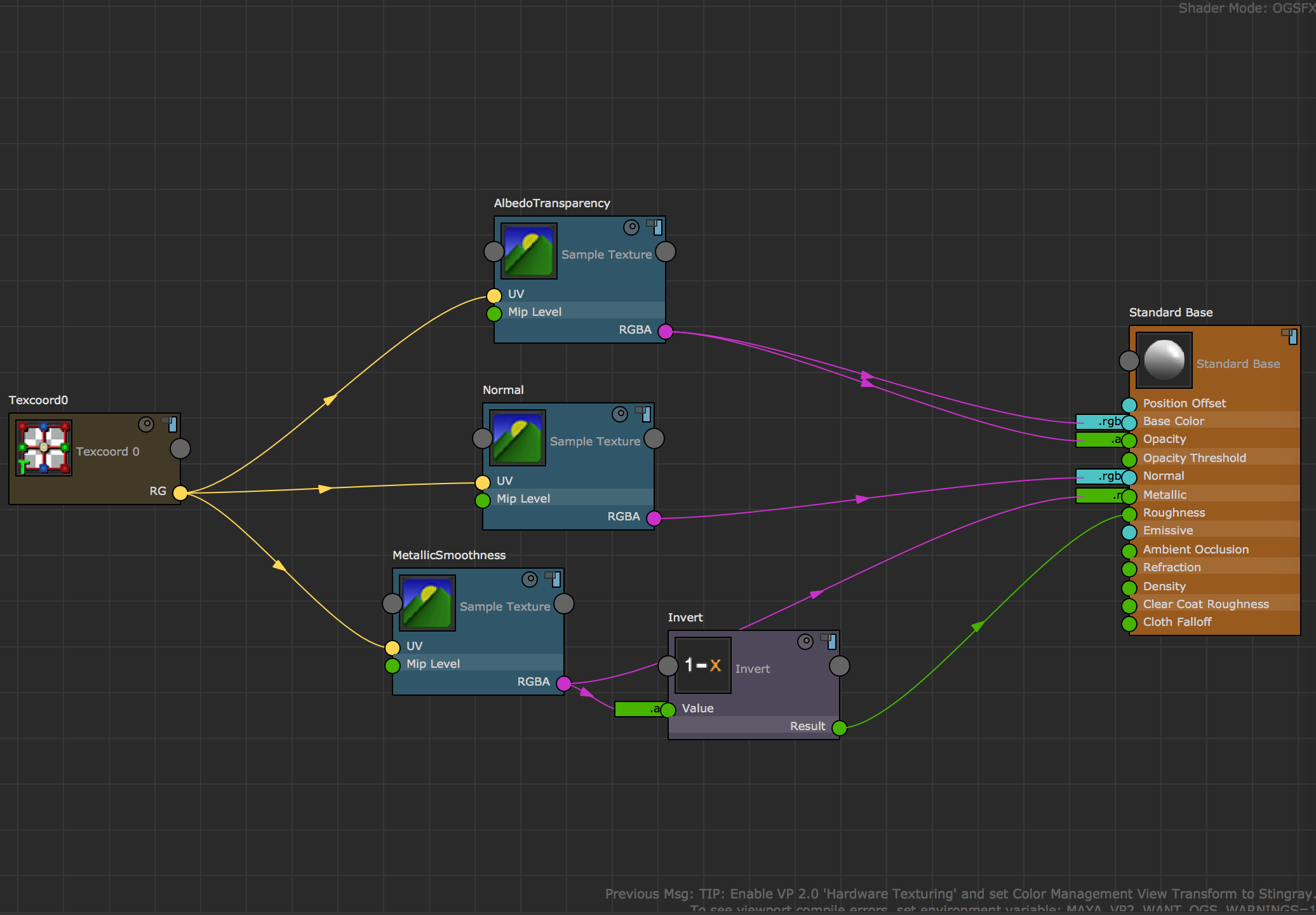
We show both quantitatively and qualitatively that our method is highly effective at various tasks such as 3D shape generation, single view reconstruction and shape manipulation, while being significantly faster and more flexible compared to recent 3D generative models.Maya 2018 | Arnold 5 In this example, the lamp shade has a Translucent material Moreover, the use of standard 2D architectures can help bring more 2D advances into the 3D realm. The generated geometry images are quick to convert to 3D meshes, enabling real-time 3D object synthesis, visualization and interactive editing. Specifically, we propose a novel method to convert 3D shapes into compact 1-channel geometry images and leverage StyleGAN3 and image-to-image translation networks to generate 3D objects in 2D space. This paper addresses a central question: Is it possible to directly leverage 2D image generative models to generate 3D shapes instead? To answer this, we propose XDGAN, an effective and fast method for applying 2D image GAN architectures to the generation of 3D object geometry combined with additional surface attributes, like color textures and normals. However it is difficult to extend this progress into the 3D domain since most current 3D representations rely on custom network components. Generative models for 2D images has recently seen tremendous progress in quality, resolution and speed as a result of the efficiency of 2D convolutional architectures.
